Artificial Intelligence and Machine Learning have become integral parts of modern technology. It revolutionizing industries and transforming everyday life. These concepts, while related, have distinct definitions and applications. Understanding AI and ML provides a clearer view of their role in shaping the future.
Artificial Intelligence and Machine Learning
Defining Artificial Intelligence
Artificial Intelligence refers to the simulation of human intelligence processes by machines, especially computer systems. It enables machines to perform tasks that typically require human intelligence, such as reasoning, problem-solving, understanding language, and learning from experience. AI can be categorized into three main types:
Narrow AI: Also known as Weak AI, this is designed to perform specific tasks, such as voice assistants like Siri or recommendation algorithms on streaming platforms. Narrow AI operates within predefined parameters and excels at its designated function but lacks general intelligence.
General AI: This hypothetical form of AI would have the ability to understand, learn, and apply intelligence across a wide range of tasks, similar to human cognitive abilities. General AI remains a subject of research and development.
Superintelligent AI: This theoretical concept involves AI surpassing human intelligence across all fields. While it’s a popular topic in speculative discussions, superintelligence is still far from reality.
AI’s applications range from natural language processing (NLP) and robotics to autonomous vehicles and predictive analytics. Its versatility makes it a cornerstone of technological advancement.
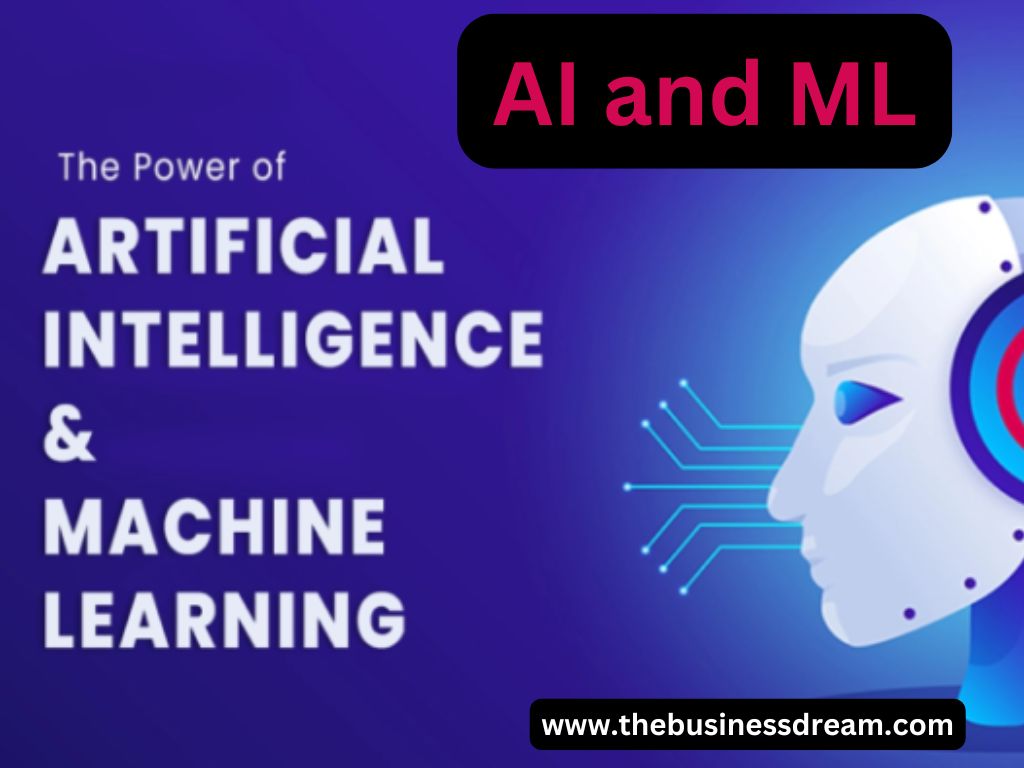
The Basics of Machine Learning
Machine Learning, a subset of AI, focuses on enabling machines to learn from data without being explicitly programmed. It leverages algorithms to identify patterns, make decisions, and improve performance over time. The core principle of ML lies in using statistical techniques to enable systems to learn autonomously.
There are three primary types of machine learning:
Supervised Learning: In this approach, the model is trained on a labeled dataset, meaning the input data is paired with the correct output. For example, an ML model might be trained to recognize cats and dogs by analyzing labeled images.
Unsupervised Learning: Here, the data is unlabeled, and the model identifies patterns and structures without explicit guidance. Clustering algorithms, often used for market segmentation or anomaly detection, are a prime example.
Reinforcement Learning: This type involves an agent learning to make decisions by interacting with an environment. Through trial and error, the agent maximizes rewards and minimizes penalties, as seen in applications like game-playing AI or robotic navigation.
ML’s capabilities are evident in diverse applications, including fraud detection, speech recognition, and personalized recommendations. Its ability to process vast amounts of data efficiently makes it indispensable in data-driven industries.
Key Differences Between AI and ML
While AI and ML are closely related, they are not interchangeable. AI encompasses a broader scope, aiming to replicate human-like intelligence, while ML focuses specifically on learning from data. In essence, ML is a tool that enables AI systems to function effectively. Understanding this distinction is crucial for appreciating their unique contributions.
Real-World Applications of AI and ML
The practical implementations of AI and ML are vast, affecting industries ranging from healthcare to entertainment. Here are a few notable examples:
Healthcare: AI-powered diagnostic tools analyze medical images, predict patient outcomes, and assist in drug discovery. Machine learning algorithms improve precision medicine by tailoring treatments to individual patients.
Finance: AI detects fraudulent activities, assesses credit risks, and optimizes investment portfolios. ML models analyze historical data to provide real-time insights for better decision-making.
Retail and E-commerce: Personalized shopping experiences, inventory management, and dynamic pricing strategies are driven by AI and ML. Recommendation systems, like those used by Amazon or Netflix, enhance customer satisfaction.
Transportation: Self-driving cars and intelligent traffic management systems rely on AI to improve safety and efficiency. Machine learning algorithms enable autonomous vehicles to adapt to real-world conditions.
Entertainment: AI generates realistic virtual environments and characters, while ML enhances content recommendations, ensuring users find what they enjoy quickly.
These examples highlight the transformative potential of AI and ML in enhancing productivity, improving outcomes, and enabling innovative solutions.
Challenges and Ethical Considerations
Despite their benefits, AI and ML pose significant challenges and ethical dilemmas. Issues include:
- Bias in Algorithms: ML models can inherit biases from training data, leading to unfair outcomes. Addressing these biases requires diverse datasets and rigorous evaluation.
- Privacy Concerns: The extensive use of data in AI systems raises questions about user privacy and data security. Transparent data practices and robust regulations are essential.
- Job Displacement: Automation powered by AI and ML threatens certain job sectors, necessitating reskilling programs and workforce adaptation.
- Accountability: Determining responsibility for AI-driven decisions, especially in critical areas like healthcare and law enforcement, is a complex issue.
Balancing innovation with ethical considerations ensures that AI and ML benefit society without exacerbating inequalities or risks.
READ ANOTHER: Important Aspects of Website Development
The Future of AI and ML
The future of AI and ML is promising, with ongoing advancements in areas like deep learning, natural language understanding, and quantum computing. Emerging trends include:
AI in Education: Personalized learning platforms and virtual tutors will transform education by catering to individual student needs.
AI for Sustainability: Machine learning models optimize energy consumption, enhance renewable energy management, and predict environmental changes.
Human-AI Collaboration: Instead of replacing humans, AI systems will augment human capabilities, leading to more effective collaboration across industries.
As these technologies evolve, fostering interdisciplinary research and maintaining ethical oversight will be critical to realizing their full potential.
Verdict words
Artificial Intelligence and Machine Learning are redefining the technological landscape. Its offering unprecedented opportunities for innovation and growth. While they present challenges, their potential to solve complex problems and improve lives is undeniable. By understanding their foundations, applications, and implications. We can better prepare for a future shaped by intelligent systems.